Dash Collector
Project Description
The Dash Collector project was a multi-semester project during my undergraduate tenure at Georgia Tech which focused on developing a system to predict the driver of a vehicle based on the driver’s driving pattern.
The first portion of the project involved the development of an iOS application to connect with the car’s OBD port, making use of the Kiwi 2 OBD2 Diagnostic Scanner. The developed application connects wirelessly with the device to access the OBD port of the vehicle, and asynchronously sends requests to the car to receive necessary information using the various PIDs described here: https://en.wikipedia.org/wiki/OBD-II_PIDs#Standard_PIDs. The application saves the data locally using Core Data, as well as sends the data to the RESTful API if the user has network access. If access to the internet is not available, the application will send the data once network access has been re-established.
Beyond the iOS application, I also developed a Web Application to collect the data from the iOS application using a RESTful API, as well as login and signup. Through the web application, various user types can be differentiated based on their credentials and give the capability of monitoring various types of users, vehicles, and thus driving styles. In addition to the aggregation of the vehicle data, the web application also leverages several machine learning algorithms to preprocess the data and train models for each user based on each of their individual driving sessions. Based on the data collected, the system is capable of making a determination of the driver based on the various drivers within the system, and thus send a notification after a specified time, identifying the expected driver as well as the accuracy of the determination.
Future work includes more data cleanup and event-based predictions on critical events like starts and stops, turns, etc.
The research continued into a focus on work with drone technologies to uniquely identify a drone by tracking its flight pattern. The research focused on creating two different types of flight signatures: the first signature is based on data collected from sensors on the drone, and a second signature based on data collected from an on-ground camera. This research is in its infancy.
Key Contributions
I had the opportunity to build the entire system, including an iOS application and web application, from the ground up. From the web application perspective, the backend was composed of Python Django and a PostgreSQL database. The frontend was built using AngularJS to display statistics, information, show progress and estimations, initialize jobs, and provide alerts to users. The system is also load balanced using AWS and Nginx to automatically scale based on the overall system usage.
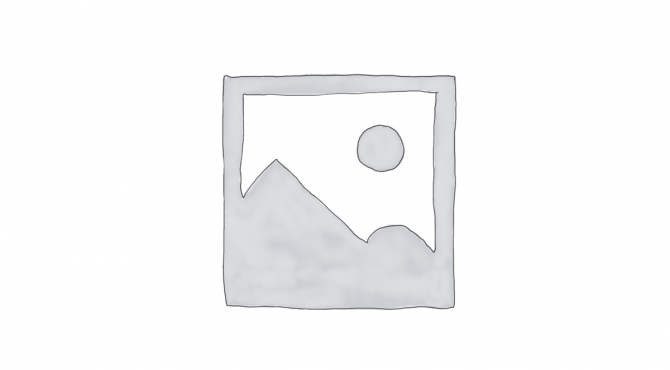
Customers/Partners
Georgia Institute of Technology, SRI International
Services Provided
Web Development, Security, iOS Development, Machine Learning
Technology
Python, Django, Scikit-Learn, Nginx, AngularJS, Objective-C, AWS